Clinical Validation at the Intersection of Mental Health and AI
In medicine and healthcare, the minimization of risk and standardization of diagnostic and therapeutic processes is of utmost importance. For this reason, each new health technology, therapy, and methodology needs to undergo a rigorous clinical validation process before entering clinical practice. Essentially, there cannot be a shred of doubt surrounding its accuracy, reliability, safety, and efficacy.With the emergence of powerful new technologies like artificial intelligence (AI), the clinical validation process has become more important than ever. Over the past few decades, we’ve witnessed the development, analysis and exploration of AI tools across various healthcare applications. However, when it comes to ensuring their clinical validity, there are additional considerations to factor in.That’s why clinical validation has been a core part of our company from day one – and we have an entire team dedicated to clinical research. In this article, we’ll break down how we’re navigating the clinical validation process as we develop an artificial intelligence diagnostic tool in the mental health space, and explain how we aim to address existing challenges in the field.
Prentice's Perspective: The Kintsugi Journey
The problem we're tackling
In the current US healthcare paradigm, mental healthcare delivery is failing. A growing mental health crisis has been brought on by several converging factors, placing greater strain than ever on already overburdened healthcare infrastructures. Almost half of the US population lives in mental health workforce shortage areas. Clinician burnout is on the rise, there's a higher patient-to-clinician ratio than ever before, with the length of healthcare encounters decreasing and the burden of documentation growing as a result.

Even when clinicians recognize that a patient is depressed or anxious (which can be extremely challenging in itself), they often only have the time and resources to address more pressing physical health concerns. As a result, less than half of individuals with mental health conditions receive treatment. The figures are even worse for vulnerable and minority populations, who are screened for depression and anxiety at much lower rates.

Kintsugi was born to tackle this enormous issue, bringing a noninvasive, quantifiable, highly scalable and objective mental health screening tool to the clinical experience that analyzes a patient’s voice for signs of depression and anxiety in seconds. Our technology surpasses the PHQ-2, a two-question questionnaire, currently the most frequently used screening tool for depression. AI-based solutions like Kintsugi can run seamlessly in the background of patient visits, providing real-time insights into a patient’s mental wellness based on their voice biomarkers, and taking no additional clinician time for interpretation.
A multifaceted process
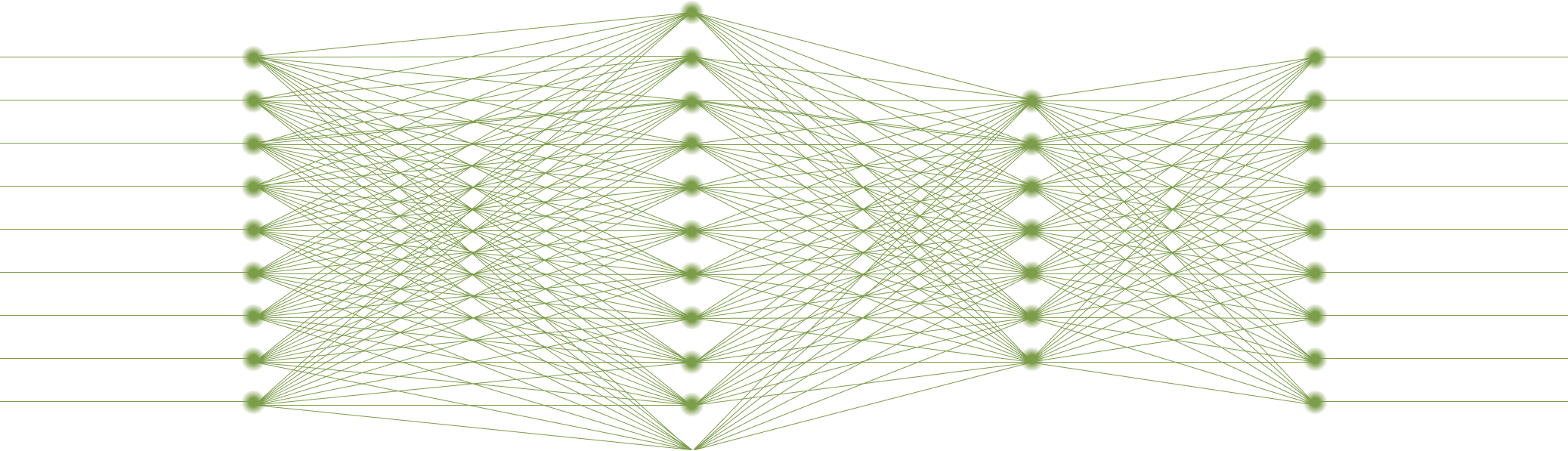
The promise of AI in healthcare stems from its ability to analyze potentially countless samples and predictably detect patterns from large sets of data. For example, while a doctor may see a few hundred to a few thousand patients throughout their career, AI models can be fed millions of data points simultaneously, helping clinicians, especially generalists, more easily uncover signs of disease that may have previously gone unnoticed in clinical practice.
With this in mind, we’ve built our proprietary machine-learning model using an enormous and growing data set. Our primary data collection source began via our consumer voice journaling application, which has over 200K downloads and users in over 250 international cities, as well as via in-house surveys. From this foundation, we have continually added data from our academic, clinical, and commercial engagements to further refine our model.
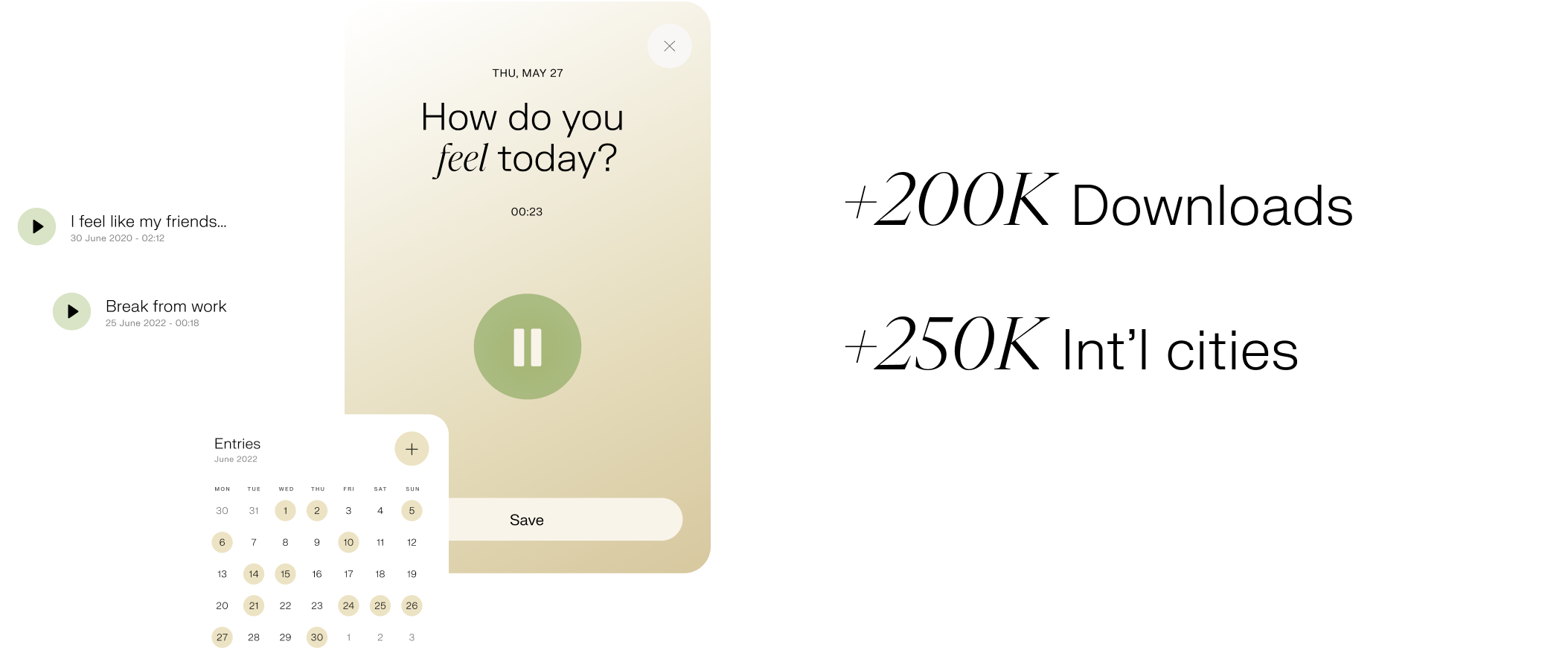
At the same time, we run clinical validation studies, whereby we test the feasibility and acceptability of our technology in real-world settings, as well as guaranteeing its safety and efficacy. These studies assess the ability of our machine learning model to identify significant signs of depression and anxiety from someone's voice. We are currently doing further validation of our technology by comparing it against industry gold standards in mental health condition diagnosis.We recently conducted a study presented at the ATA which showed Kintsugi's machine learning model was able to detect signs of depression in a reserved test set of 340 severely depressed patients with a sensitivity of 0.91 with no false positives. The results suggest that this technology can expedite and improve depression detection and screening, especially for those patients with the most severe depression and in greatest need of medical intervention.

Additional challenges to overcome
In the clinical research process, carrying out research in an ethical way is just as essential as designing rigorous studies. We are testing our technology on real patients and alongside real clinicians, so every measure must be taken to prevent harm and ensure safety. An extra challenge that has emerged, particularly in the post-COVID era, is that clinical studies are often carried out remotely – especially when working with digital health tools.
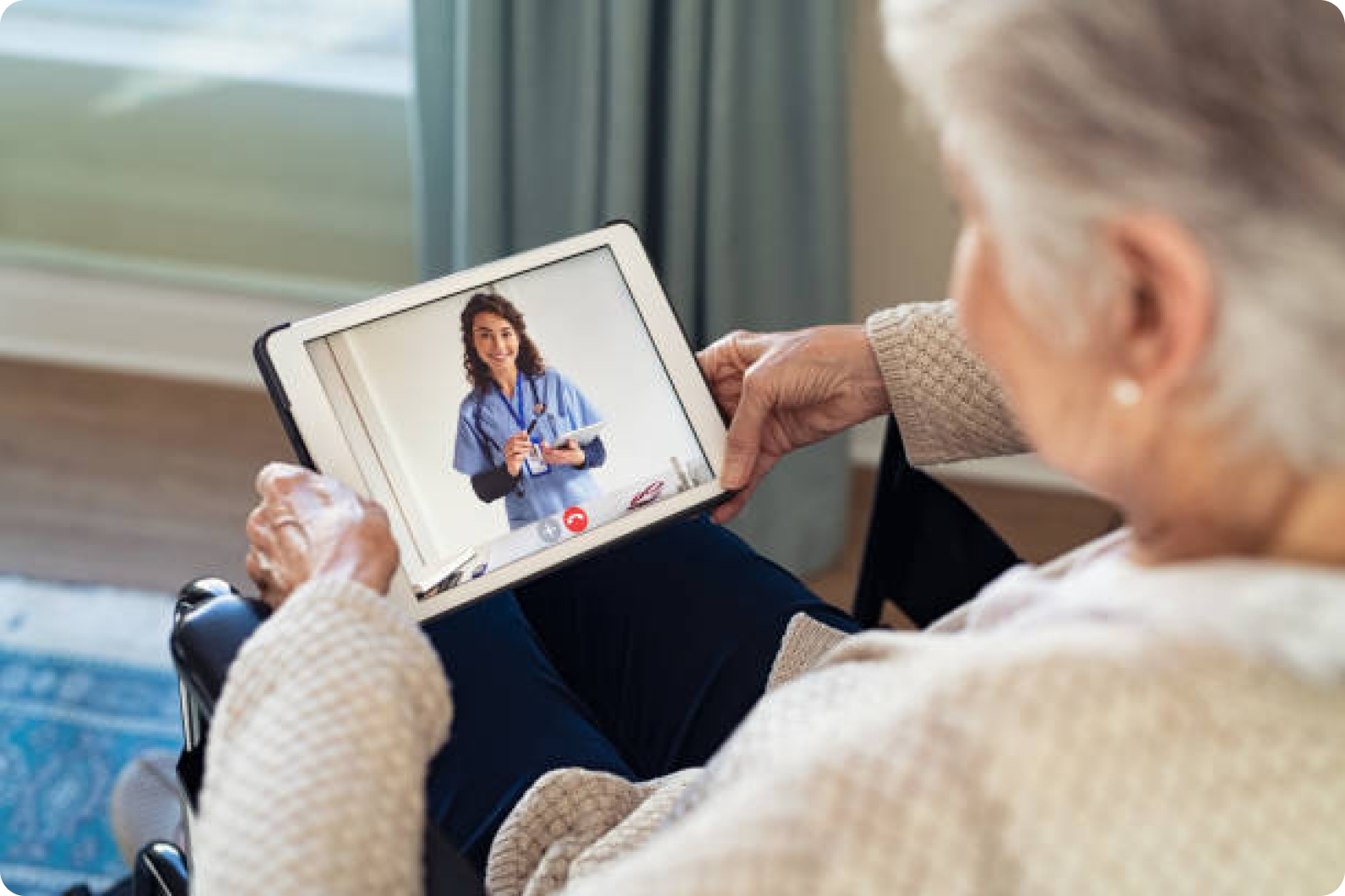
Without face-to-face contact, it can be difficult to obtain patient and clinician buy-in. Gaining the trust of our patients and clinicians is a critical component of our research process, and this is especially important for our vulnerable minority patient populations, who can sometimes be disenfranchised and less trusting of our healthcare system. Our aim is to collect as diverse a dataset as possible to ensure our models are as representative as the US, and we design our studies with this goal in mind.
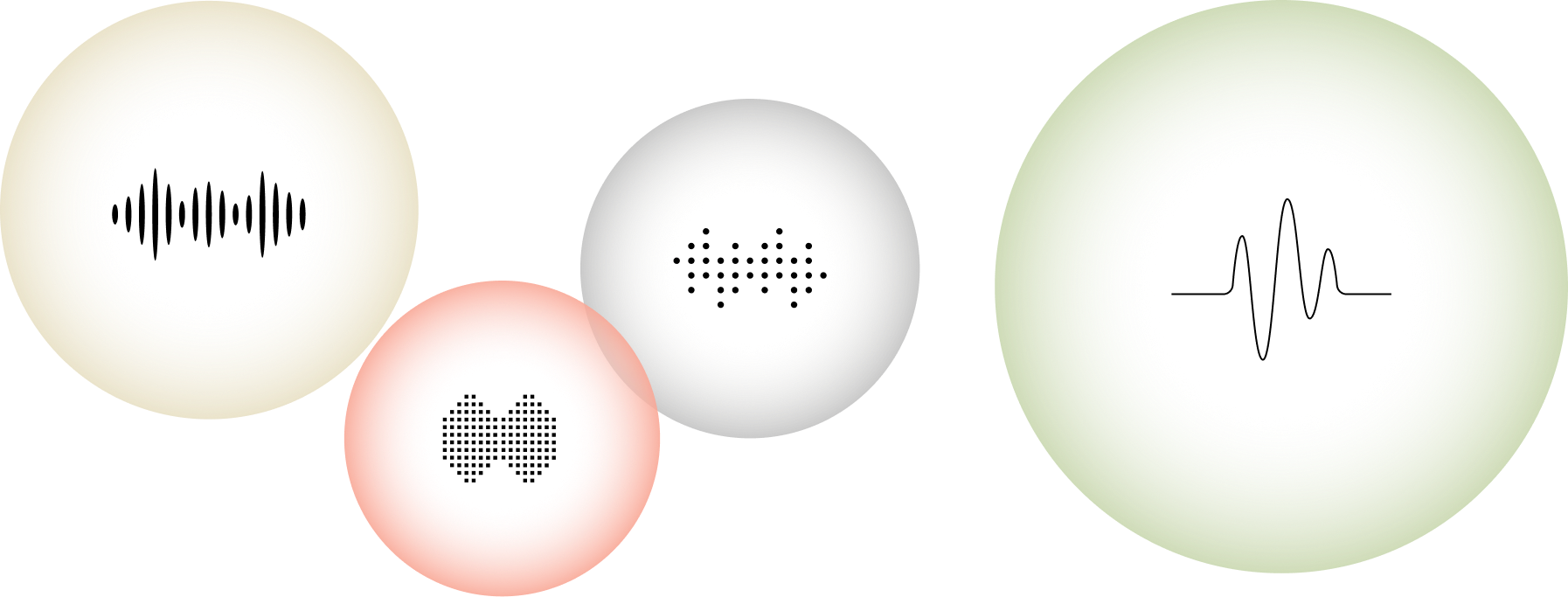
One approach we’ve taken is to launch targeted recruitment campaigns as well as reach out to clinicians and providers who work with specific minority and geographic populations. This has allowed us to represent people who may not have access to mental health care as well as mimic a patient population that is representative of the nation and globe. In doing so, we can improve overall model performance and generalize insights, while at the same time removing bias that has historically led to under-diagnosis in vulnerable populations. In addition, since we’re working with cutting-edge technology that is already transforming society, we have to take extra care to accurately log all our methods and findings, as well as the potential risks and limitations. We also need to keep a clear record of the flow of information from our tool in a clinical setting, how patients and clinicians interact with it, and how the clinician uses it to make decisions in their clinical workflow. With this in mind, we share the results of all our clinical studies in open-access journals, so that other members of the scientific community can explore and build on the knowledge we generate in the field.
How this translates back to the clinic
Ultimately, we want to bring a clinically-validated biomarker to the mental health space: something that is currently lacking in the field (and thus holding back care). We know that biomarkers directly improve patient care. Patient concerns and specific care needs are too often ignored when there are no existing biomarkers of a disease and its severity, as we have seen for now, well-documented conditions such as chronic fatigue syndrome and more recently long-COVID.

In many ways, the care for mental health conditions has mirrored that for chronic fatigue syndrome. An estimated 2.5 million Americans suffer from chronic fatigue, but in the absence of biomarkers, it can take years to receive a diagnosis, meaning patients are left to fend for themselves rather than receiving care. The same is often true for patients suffering from mental health conditions, and on top of this, there is the additional challenge of stigma preventing people from speaking up in their moment of need.
What does the future hold?
Despite the challenges, we remain steadfast in our goal of improving the delivery of mental health care in the US and beyond. With each research question that we address, we have found that several new ones emerge, and thus, we are always on the lookout for commercial partners who are equally curious and enthusiastic about exploring the untapped potential of AI in healthcare. If you’re interested in learning more and working with us, we would love for you to get in touch at hello@kintsugihealth.com. Our most fascinating joint projects to date have begun with an email.
Join our mailing list for regular updates from Kintsugi
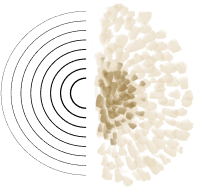