Machine Learning Detects Signs of Depression From Speech Samples in Individuals Self-Reporting Severe Depression
March 22, 2023
This cross-sectional study was conducted to determine the feasibility of using machine learning to detect signs of depression from speech samples, with the aim of identifying at-risk patients for screening in virtual care. Participants provided voice responses to the prompt “how was your day?” and self-reported PHQ-9 scores.
Kintsugi's machine learning model was able to detect signs of depression in severely depressed patients with a sensitivity of 0.91 and no false positives. The results suggest that this technology can expedite depression detection and screening, especially in virtual primary care settings where formal screening may not always occur.
Click here to read the full abstract.
Join our mailing list for regular updates from Kintsugi
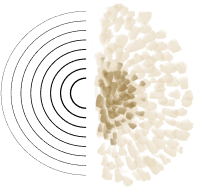